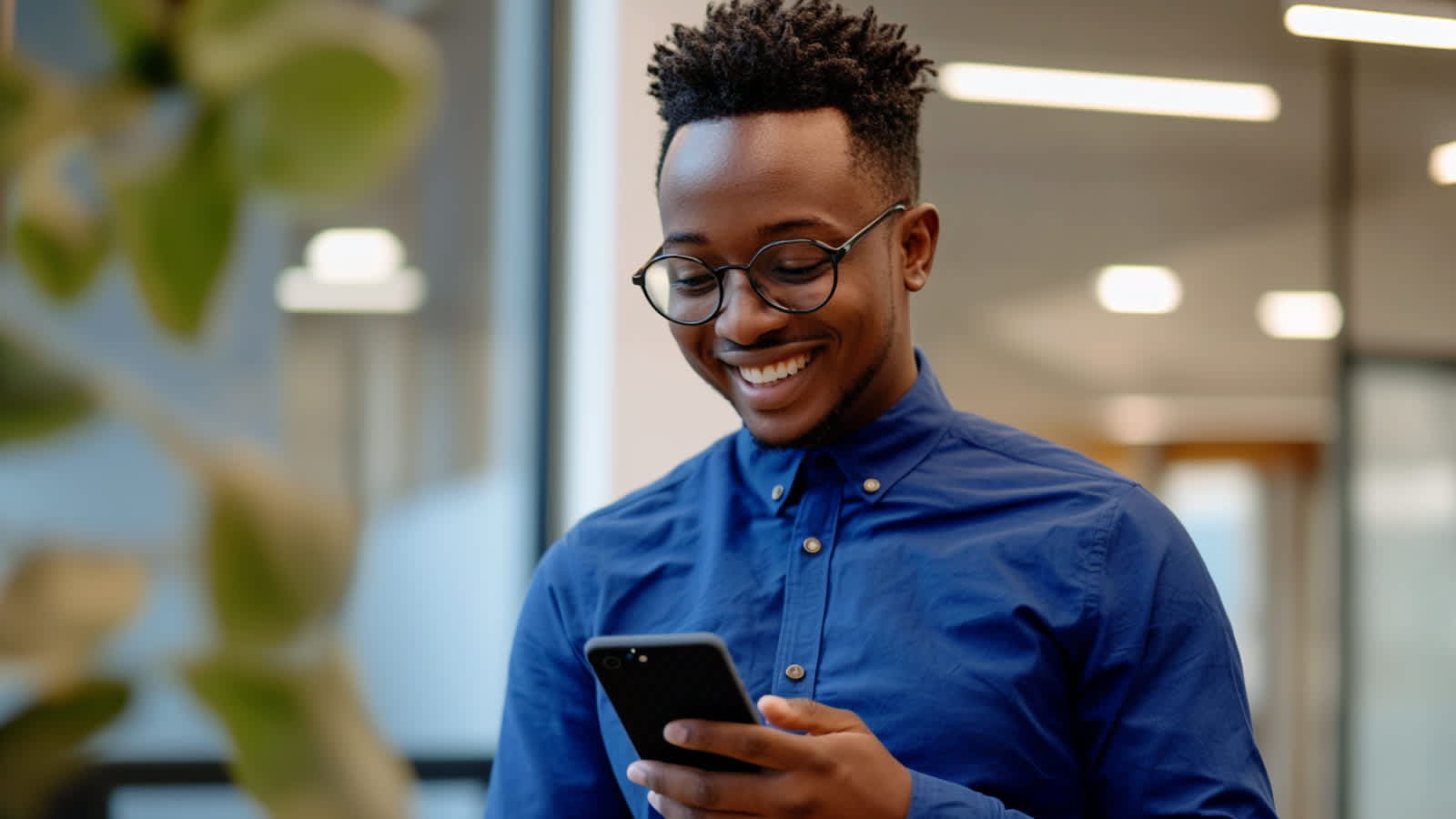
The Data Science team at Job&Talent is responsible for bringing cutting edge AI intelligence to the products and offerings of the company, both in terms of customer facing front-end features in the app and more under-the-hood improvements that future-proof our infrastructure. In addition to conceiving, developing, testing and deploying AI models, the Data Science team takes an active role in bringing thought leadership around ethical and responsible use of AI in a commercial setting that leads to improved experience of clients, workers and internal stakeholders alike.
It’s said that data is the new oil of the corporate economy. In today’s era of analytics and AI, the truth of this is being felt across the board, and the staffing industry is no exception. Advanced tools and techniques have become integral to the workflow of every competitive staffing company, from AI-based hiring bots to machine learning applications that optimize workforce planning and management.Â
AI applications are naturally data hungry, and in AI and machine learning, the role of data is twofold. First, in order to train private, custom models for application in product and operations, a significant volume of curated and formatted data is required to pass through machine learning algorithms (such as tree based classifiers or neural networks).Â
Secondly, data is required at the application stage, called ‘inference’ in machine learning vocabulary, to run predictions on trained models and extract forecasts and recommendations. Due to this heavy reliance on data in such applications, a legitimate concern is to ensure data protection, privacy and security. How can we harness the power of data responsibly while safeguarding the sensitive information it contains?
Navigating data protection best practice
In the European Union, the General Data Protection Regulation (GDPR) lays out the legal framework for data protection. But what does it actually mean in terms of how data professionals handle and build AI applications in their day to day work?
At Job&Talent, we see data handling through several different lenses. For the purpose of AI systems, we focus on two types of data: data for training and data for inference, corresponding to the two modes of use described above. It is important to note that there are other ways to classify data, for example the view of data in motion and data at rest—a paradigm more commonly used by data engineers.Â
Responsible data collection and training
For data training, the process starts with responsible data collection. We strive to collect data that is useful for our models, masking or removing any information that does not directly fulfil this purpose. Our AI systems are designed to avoid collecting or using personal data (PII) or identifications, such as names, phone numbers, email addresses, as they often have little value in descriptive or predictive analytics. Instead, we rely on randomly assigned unique numerical identifiers, like customer IDs, to index data.Â
Additionally, while preparing data for training, we are careful to exclude any descriptors that are private or potentially discriminatory in nature, such as age, income bracket, or ethnicity, even if they may have predictive value. This practice allows us to prevent potentially biased recommendations from the models down the line, and reduces the risks of storing sensitive data during the training process.Â
Ensuring security during inference
At time of inference, where models are applied in real-time or near-real-time within a production environment, the situation is more critical. In such an environment, there is a direct interface to users (clients and workers in our case) whose private information may be involved. To mitigate risks, we implement robust security protocols and layers in our AI services, such as strict authentication, input validation, and whitelisting.Â
These practices protect our systems from unauthorized requests, prevent injection of malicious code in services that cause them to leak unintended information, and only keep internal responses restricted to known and trusted services. Additionally, we follow best practices such as encrypting data in motion when it is being transmitted between services, discarding unnecessary inputs after use (zero data retention), and validating outputs to ensure they contain only necessary information in contracted formats.Â
Responsible use of generative AI modelsÂ
Not all models are trained, particularly in the era of Generative AI, where commercial large language models (LLMs) are increasingly used for business applications. When using these models, responsible data usage takes on a different flavor.Â
GenAI models are mainly used in inference mode, and at Job&Talent we use them in two ways. For commercial third-party models such as OpenAI or Anthropic AI, or platforms like AWS Bedrock or MS Azure AI, we ensure that the terms of usage and data privacy align with our own standards of commitment to user data protection. We also ensure a zero data retention policy so that none of the inputs can be stored or used for training purposes.Â
When using open source LLMs—such as Meta’s Llama3, or Mistral AI’s Mixtral 7B model— Although the model is not trained by us we still run comprehensive testing to identify and mitigate common problems with GenAI such as hallucinations, inappropriate outputs, biased results, or incorrect calculations. The research community is active in coming up with definitive benchmarks to ensure safety and compliance for LLM usage, and staying updated with these standards is critical for AI adoption success.Â
The human responsibility in AIÂ
In the end, current AI technology is not a self-governed independent entity. As AI practitioners and engineers, we are responsible for training, securing, and verifying these systems in our applications. A thoughtful, user-centric approach is essential to build trust and reliability in these AI systems, which are already rapidly transforming the way we work and the value we bring to our users.
Interested in responsible AI? Read more about how AI can enhance the human connections in staffing.